Risk management and return maximization are critical for individuals, enterprises, and organizations alike in the complex and unstable financial world of today. With its data-driven strategies and insights, financial analytics has become a potent instrument for achieving these objectives. It enables decision-making based on solid information. This article will examine the field of financial analytics, including its application to risk management and its ability to maximize profits.
Understanding Financial Analytics
Financial analytics is the process of analyzing, interpreting, and predicting economic trends, opportunities, and hazards using data and mathematical models. It makes use of a variety of tools and approaches to extract valuable insights from enormous amounts of financial data, assisting stakeholders in making wise decisions in areas like investing, managing portfolios, and financial planning risk management.
Elements of Financial Analytics
Financial analytics is a crucial field that leverages data analysis techniques to inform financial decision-making and strategy. It encompasses a variety of methods and tools to evaluate financial performance, forecast future outcomes, and guide investment and Risk management. Here are the core elements that comprise financial analytics:
Data Gathering
Financial analysts acquire information from a variety of sources, such as market information, economic indicators, corporate financial reports, and more. The basis for the analysis is this data.
Data Processing
Raw data is cleansed, categorized, and turned into an analytic-ready format. This process frequently entails dealing with missing data, eliminating outliers, and guaranteeing data consistency.
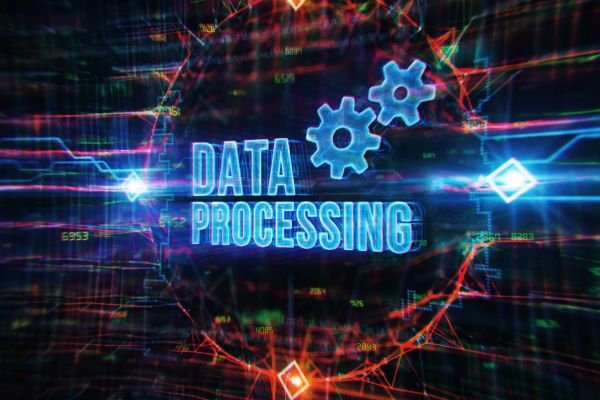
Data Analysis
The processed data is subjected to a variety of statistical and mathematical approaches to reveal correlations, patterns, and insights. Analyses like this include identifying trends, assessing performance, and assessing risks.
Modeling
Financial analysts develop mathematical models that recreate various scenarios, project future results, and evaluate potential hazards. Based on the individual objectives, these models can be basic correlation analyses or more complicated ones like Monte Carlo simulations.
Reporting
The results of the analysis are compiled in thorough reports that give stakeholders advice or new information.
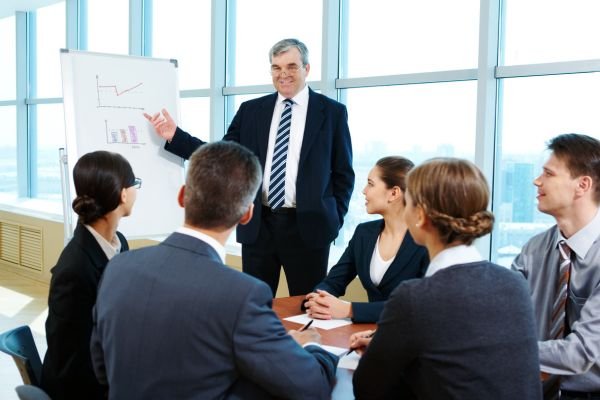
Role of Financial Analytics in Risk Management
A key component of risk analytics management. Here are some ways that financial analytics aid in effective data analytics risk management:
Risk Identification
The complete identification and evaluation of risks is one of the main purposes of financial analytics. Financial analysts can identify possible risks in a number of domains, including market risk, operational risk, credit risk, and liquidity risk, by examining historical data and market trends. It is essential to identify these hazards to create efficient financial risk management models.
Risk Evaluation and Measurement
Organizations can clearly grasp the potential impact and likelihood of unfavorable events thanks to the quantification of risks made possible by financial analytics. The scope of financial troubles is measured and evaluated using a variety of risk indicators and models, including Value at Risk (VaR) and Stress Testing. These criteria assist companies in deciding how much capital to set aside to offset probable losses.
Portfolio Diversification
A key tactic in risk control is portfolio diversification. Effective portfolio diversification requires the use of financial analytics, which is crucial for investors and fund managers. Financial analysts can build portfolios that lower overall risk while maximizing returns by examining correlations between various assets and asset classes.
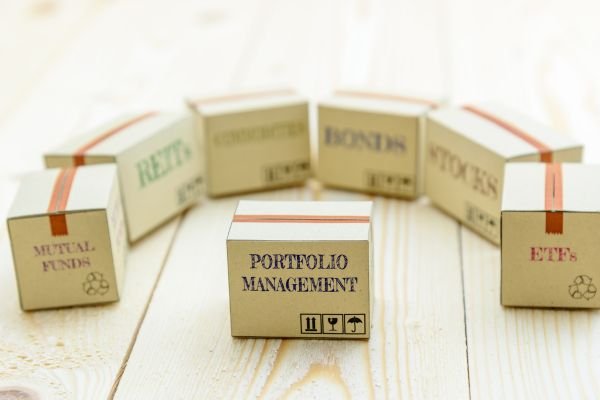
Stress Testing
Risk management must include stress testing, notably in financial and banking services. Institutions can examine the durability of their assets, portfolios, and capital reserves under challenging circumstances by simulating extreme scenarios and using financial analytics. Organizations can find weaknesses and implement preventive actions with the help of this proactive strategy.
Regulatory Compliance
Financial analytics helps firms in a time of strict economic laws, such as Basel III for banks, to comply with legal requirements. It helps with risk-weighted asset calculation, capital adequacy measurement, and ensuring that institutions retain sufficient resources to meet regulatory requirements.
Maximizing Returns through Financial Analytics
While risk management is crucial, financial analytics are as important for maximizing returns. Here’s how it works:
Making Investment Decisions
Financial analysts employ data analytics to find investment possibilities that match the risk appetite and return goals of a company. They can discover undervalued assets, growth prospects, and market trends that could produce higher profits by doing in-depth research and analysis.
Performance Assessment
It’s critical for maximizing returns to measure the performance of assets and portfolios. Investors can use financial analytics to monitor the progress of their assets over time, evaluate how they perform in comparison to benchmarks, and modify returns.
Predictive Modeling
Organizations can predict future economic consequences with the aid of predictive modeling, a crucial component of financial analytics. This skill is especially useful for seeing market patterns, forecasting asset price changes, and making wise investment choices that optimize returns.
Algorithmic Trading
The emergence of algorithmic trading—where computers employ advanced analytics to execute deals at ideal times and prices—is a result of financial analytics. This strategy can take advantage of market imperfections and react quickly to shifting market circumstances, thereby maximizing returns.
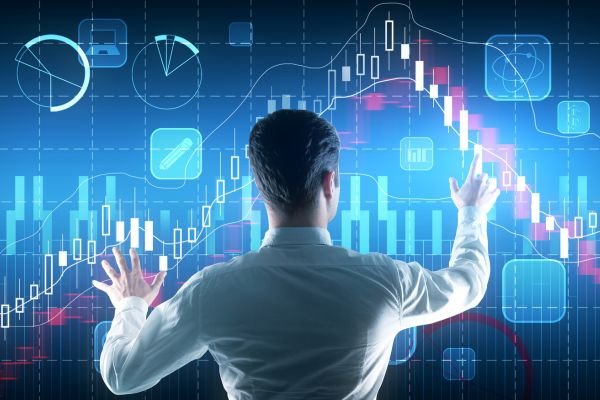
Risk-Return Balance
Enterprises may balance risk and return with financial analytics. Investors can choose their portfolios wisely and obtain the required level of returns while effectively controlling risk by evaluating the risk-return trade-off.
Tools and Techniques in Financial Analytics
A variety of technologies and strategies are available to execute financial analytics effectively, including:
Software for Statistical Analysis (SAS)
SAS is an effective tool for modeling and data analysis. Financial analysts can do intricate computations and build predictive models using its wide set of statistical algorithms.
Spreadsheets and Excel
Excel’s convenience and adaptability make it an effective tool for financial evaluation. Financial experts utilize it for data processing, modeling, and reporting.
R and Python Programming
Python and R are two well-liked programming languages for data analysis and machine learning. It is one of the most futuristic data analytics trend that provide huge libraries and programs designed specifically for financial analytics.
Data Visualization Tools
Interactive visuals and dashboards may be made with the help of programs like Power BI, Tableau, and QlikView, which improve data exploration and collaboration.
Artificial Intelligence and Machine Learning
Fraud detection, Predictive Modeling, and algorithmic trading are just a few of the jobs that use machine learning algorithms in financial analytics.
Risk Control Software
Organizations may assess, monitor, and mitigate a variety of risks with the use of specialized risk management tools like RiskWatch and RiskMetrics.
Financial Modeling Software
MATLAB and Mathematica are two examples of software used for designing and simulating financial models, particularly for complicated risk assessments.
Challenges in Financial Analytics
Although financial analytics has many advantages, it also has a number of drawbacks:
Data Accuracy And Quality
In financial analytics, data quality and accuracy are crucial. Data that is inaccurate or lacking might provide poor analyses and incorrect conclusions.
Data Privacy and Security
Data security and privacy are major issues since financial data frequently contains sensitive information. Respecting data privacy regulations is essential.
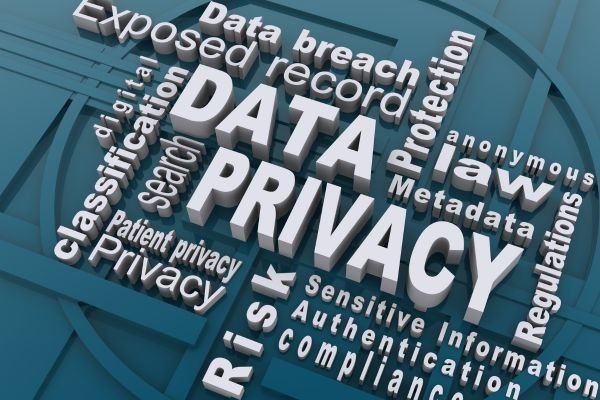
Model Risk
Financial analytics models simplify reality, and as a result, their underlying assumptions might not always be true. Model risk occurs when projections made by the model differ from the final results.
Regulatory Conformity
It might be difficult to comply with regulatory requirements because they are always changing. Financial institutions need to keep current and modify their analytics procedures as necessary.
Communication and Interpretation
It might be difficult to interpret complicated analytics data and successfully communicate them to stakeholders. It is vital to make sure that decision-makers are aware of the analysis’ implications.
The Bottom Line
In today’s chaotic market, financial analytics is essential for managing risk and maximizing returns. It aids in decision-making, risk detection, and plan improvement for both businesses and people. Using statistical analysis to manage risk and maximize returns can assist companies, financial institutions, and individual investors to succeed financially.