In the rapidly evolving landscape of data analytics, the integration of Generative AI is reshaping the way businesses derive insights and make decisions. With the ability to generate, analyze, and interpret vast amounts of data, Generative AI is not just an add-on but a game-changer for any data analytics agency. Companies leveraging this technology are experiencing increased efficiency, deeper insights, and automated decision-making capabilities that were once thought impossible.
This blog explores how Generative AI is revolutionizing data analytics, the benefits it brings to businesses, and what the future holds for this transformative technology.
Understanding Generative AI in Data Analytics
Generative AI refers to artificial intelligence systems that can create new content, such as text, images, and even synthetic data, based on learned patterns from existing datasets. Unlike traditional AI, which mainly classifies or predicts based on historical data, Generative AI can generate entirely new data points, fill in missing information, and simulate different business scenarios.
For a data analytics agency, this means moving beyond traditional analytics and embracing AI-driven automation, insights, and decision-making capabilities.
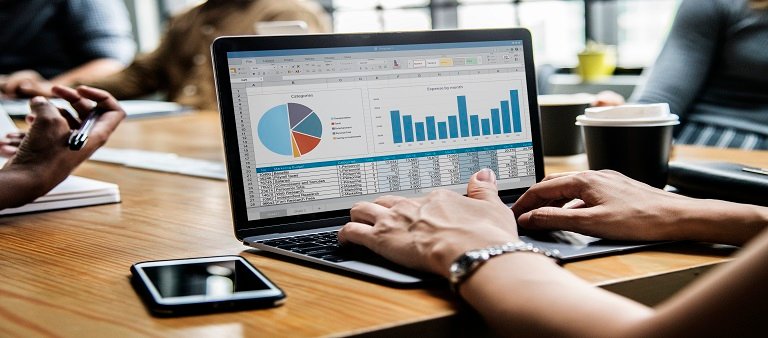
Key Ways Generative AI is Transforming Data Analytics
1. Automating Data Processing and Cleaning
Data cleaning and preprocessing are often the most time-consuming tasks in data analytics. Generative AI can automate:
- Identifying and correcting errors in datasets
- Filling in missing values with intelligent predictions
- Standardizing and categorizing unstructured data
This allows analysts to spend less time on data preparation and more time deriving valuable insights.
2. Enhancing Predictive Analytics
Traditional predictive analytics relies on historical data to forecast future trends. Generative AI takes this a step further by:
- Generating synthetic data to improve model accuracy
- Simulating potential future scenarios based on different variables
- Providing enhanced risk assessment and decision-making support
Businesses can use these capabilities to make more informed strategic decisions, reducing uncertainties and optimizing operations.
3. Automating Report Generation and Data Visualization
Creating detailed reports and data visualizations can be resource-intensive. Generative AI can:
- Generate dynamic, real-time reports based on updated datasets
- Create interactive dashboards and visualizations
- Summarize complex data insights into understandable formats
This enables businesses to get real-time insights without the need for manual report creation.
4. Natural Language Processing (NLP) for Data Interpretation
A significant challenge in data analytics is making insights understandable to stakeholders who lack technical expertise. Generative AI, powered by NLP, can:
- Convert complex data points into easy-to-read summaries
- Answer questions about datasets in natural language
- Generate automated business reports with actionable insights
For example, a data analytics agency can provide clients with AI-generated summaries of their business performance, making data-driven decision-making more accessible.
5. Advanced Anomaly Detection and Fraud Prevention
Identifying irregularities in data is crucial for fraud detection and risk management. Generative AI enhances this by:
- Detecting patterns and outliers that traditional models might miss
- Generating synthetic test cases to validate fraud detection models
- Continuously adapting to new fraud techniques through machine learning
This is particularly useful in industries like finance, healthcare, and cybersecurity, where anomaly detection is critical.
6. Personalized Customer Insights and Recommendations
Understanding customer behavior and preferences is key to improving user experiences. Generative AI helps businesses by:
- Analyzing customer interactions and generating personalized insights
- Predicting future customer needs and preferences
- Automating the creation of personalized marketing campaigns
For example, an e-commerce company working with a data analytics agency can use AI-generated insights to tailor recommendations for individual customers, boosting sales and engagement.
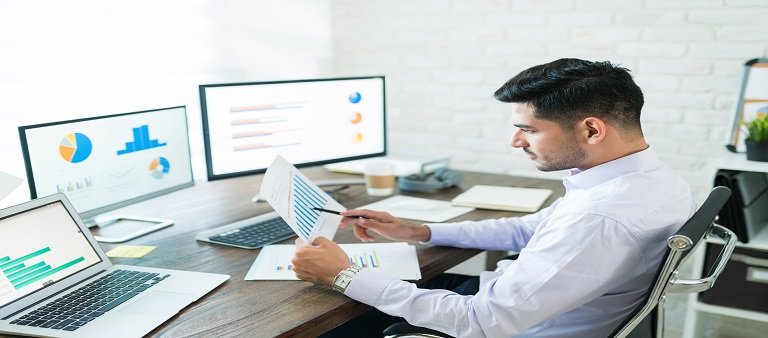
Benefits of Using Generative AI in Data Analytics
1. Increased Efficiency and Cost Savings
Automating repetitive tasks allows businesses to allocate human resources to more strategic activities, reducing operational costs and increasing efficiency.
2. More Accurate Insights
Generative AI can analyze vast datasets with high precision, reducing human error and improving the accuracy of insights.
3. Faster Decision-Making
With real-time analytics and AI-driven reports, businesses can make informed decisions much faster, staying ahead of the competition.
4. Enhanced Scalability
AI-powered analytics can handle massive datasets and scale effortlessly as a business grows, making it a valuable asset for any data analytics agency.
5. Better Risk Management
With advanced anomaly detection and predictive analytics, businesses can identify potential risks before they become major issues.
Challenges and Considerations
Despite its advantages, integrating Generative AI into data analytics comes with challenges, including:
- Data Privacy Concerns: AI requires access to large datasets, raising privacy and compliance issues.
- Bias in AI Models: If training data is biased, AI-generated insights may also be biased, leading to misleading conclusions.
- Implementation Costs: Initial setup and training of AI models require significant investment.
- Need for Skilled Personnel: Organizations must have the right talent to manage and interpret AI-generated insights effectively.
The Future of Generative AI in Data Analytics
The adoption of Generative AI in data analytics is expected to grow significantly. Future advancements may include:
- AI-driven self-service analytics: Allowing non-technical users to generate insights with minimal effort.
- More explainable AI models: Enhancing transparency in AI-generated analytics.
- Integration with IoT and real-time data sources: Providing even more accurate and up-to-date insights.
- Ethical AI governance frameworks: Ensuring AI-driven analytics are fair and unbiased.
Conclusion
Generative AI is revolutionizing data analytics by automating processes, enhancing predictive modeling, and providing deeper insights. For a data analytics agency like Eaglytics, leveraging this technology can lead to better decision-making, improved efficiency, and a competitive edge in the market.
As AI continues to evolve, businesses that embrace Generative AI-driven analytics will be better positioned to thrive in the data-driven economy. The future of data analytics is here, and it’s powered by AI.